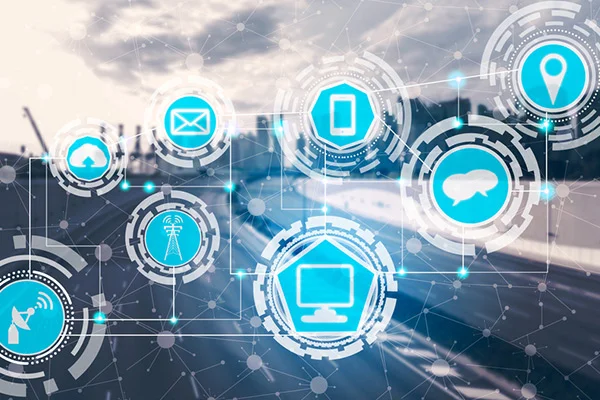
By Swanand Javadekar
Preface
The automotive industry has been facing a daunted set of challenges with upcoming connected cars, autonomous driving, and electric vehicles. It is an opportunity to differentiate for the right minds by bringing the right mix of solutions to the customer and enlightening them with more intelligent products. The following paper highlights the association of technology trends to design connected products and build efficient ecosystems for execution. Some of the aspects discussed are
- Anticipate customer needs and move from classical design mindset (collaborative designs)
- Mechanical to mechatronics (CAD to AR)
- How products are used in the field to differentiate and competitiveness of products (Insights)
Trends
There are various ways technology is changing product outlook, following are some of the exciting trends influencing product design and development cycle:
- For many years, the typical development cycle for automotive was @ 3-4 years. With the advancement of CAD and other related tools, the same has been reduced to 1.5-2 years. Even In such a scenario, the designer still has to wait for actual testing results and production cycle to get the product performance feedback. The designer can work on a live project with a feedback loop system with advanced software and sensors. For the newly launched car model, the designer will swiftly get feedback to implement optimization changes. In contrast, the older model performance can be tuned based upon the much realistic availability of load data.
- The designer will be working to build more customer-centric automotive models. He will be able to assess various patterns based on driving habits. This pattern will help him adjust, manage and tune various critical auto components to performance needs such as powertrain and engines.
- Data blending will become a more significant challenge as the designer receives and reviews data from various sources. Apart from the traditional data sources such as design, CAD data, validation, he will also collect/collate additional analytical data from sensors towards assembly performance, failure prediction, and vehicle running data. The appropriate data analytics tools need to be used for the correct outcome to be incorporated as the design parameters.
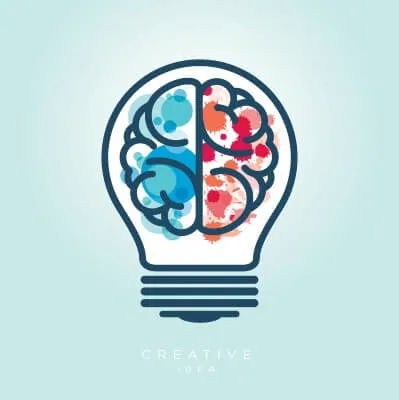
Smart Products: 4 promoting factors
Today, Smart Products have become one of the integral parts of our life. It changes the way we use products and generates new business models. This paper details layout developed to build intelligent products and discusses the contribution and trends in each sector. The discussion is limited to product design and development and not extended to manufacturing 4.0.
In a connected environment, smart products use the basic engineering data in conjunction with loT (Internet of things) / AR (Augmented Reality), embedded systems, and data analytics to provide better insight to the user and a machine manufacturer. It uses operating data from the equipment and uses a feedback loop effectively to predict various functional parameters related to product performance.
Designing smart products
As a designer who builds innovative products, he uses various advanced tools such as MBD (model- based engineering) and DEM (differential element method) to generate better insight into component behavior.
Digital twin, which generates buzz across the engineering community, is a virtual replica of a product containing representative mechanical, electrical, electronic, and performance configuration information. The digital twin is not new, as the design community is already using various CAD, CAE, and CAM tools for the past few years. However, it has witnessed changes in the ability to collect, collate and analyze big data, work towards finding trends, anomalies and use the feedback loop back to design context to make it robust. Building digital twin also leads to effectively monitoring data, leading to building newer business models.
Simulation is also one of the data-driven tools extensively used to analyze components, from simple durability to complex crash simulation. With higher computing power, the data handling capacity has been increased, as it can handle complete vehicle analysis compared to component level validation. Today, ROM (reduced-order method) based models have been used, which are machine learning solutions for reducing the size of a data set while preserving the essential parts of the information contained within that data. Such an approach now supports the user to analyze the components for rapid execution, reducing the total number of runs. There are various methods for which data analysis techniques are used: fault detection, predictive maintenance, statistical monitoring, real-time crash, and safety.
Designing connected products: AR/VR
Today AR/VR is playing a significant role in automotive product design and development. Typically, AR/VR can be extensively used for design and development, manufacturing, marketing, training, and servicing. More usage of these techniques is applied towards manufacturing and marketing, but the practice of product design is on the rise. Someone can effectively use these techniques used for design reviews and revision comparison. With the latest external devices such as hololens available in the market, the user can get an immersed view of the design for detailed assessment.
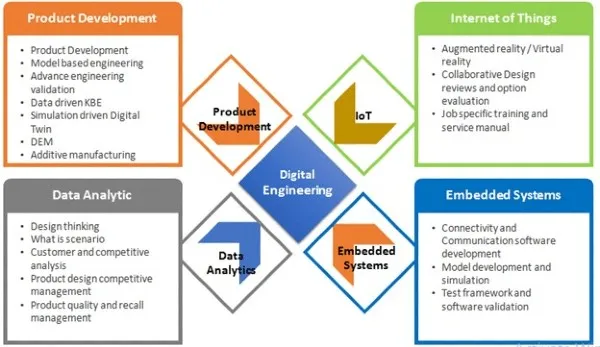
Designing Embedded products: ADAS
It’s exciting to note how these four verticals complement each other for product feature enhancement. Let’s take the example of embedded system / ADAS (advanced driver-assisted systems). We have seen that, typically, engineering simulation has been used for product development and digital twins, but the usage can be extended towards ADAS development. Some of the scenarios where validation tools can support to improve product performance understanding are semiconductor simulation (reliability analysis of Printed circuit board, energy consumption), sensor simulation (radar pattern simulation, placement of sensors compared to signal integrity), and driving scenario (software algorithm modeling simulation)
Designing insightful products
We know that Data analytics tools are effectively used for supply chain optimization, marketing mix analysis, user and dealer satisfaction, and customer behavior analysis. How can it be effectively used for a designer to view insight at an early stage?
Today product designers are facing challenges towards converting data to actionable insights. The designer will work on three types of data, design data (based upon engineering calculations), test or proving data (standard vehicle test data), and real-life running data (received via various sensors loaded at designer vehicle test points). Multiple data analysis tools/algorithms will support to decode the data effectively and will support designer to take early decisions such as component failure prediction, feature management (leads to customization of platforms).
Getting Act Together
As mentioned in the above column, various technologies work seamlessly to build a better and more innovative product. Let’s discuss a few examples of how companies use a combination of technologies to build a newer customer experience.
Design proposal selection
Today Tier1 suppliers are interacting with OEM to select their various design proposals. With AR/hololens, the supplier can offer a better immersive experience to the customer. It also helps end customers select design proposals much swiftly, saving time and money. For example, automotive interior tier 1 suppliers can envision and demonstrate “Instrument panel” fitments within the car environment to OEM’s. With changes in color scheme, shading, feature recognition, the end customer can envisage effectively for better selection.
Light-weighting
- lightweight material construction – Reinforced @ unreinforced plastic/sheet molded composites (SMC)
- Structural lightweights – tailored welded blanks/profile and tubular structure
- optimized production process – reduced no of weld spots, rivet/light joining techniques
In summary
Automotive product design has expanded beyond CAD, and advanced tools have been implemented in the early stage of the design cycle. It assures various benefits to designers such as better understanding of product behavior, customer-centric innovative design, and shortens design cycle, saving time and money.